Chapter 3 AI and Innovation
Technological change and innovation have long been recognized as crucial drivers of economic growth. Today this necessity became ever more urgent to face the challenges of the post-pandemic recovery and climate change. More certain is the extent to which a specific technology will have the desired effect, but there is a consensus on the fact that the more sectors will be invested by change, the deeper the changes in the economy will be. Since prediction technologies are a fundamental element of every activity, AI technologies are configuring as a source of profound transformation both in industry and research setting, possibly changing how innovation itself take place.
Throughout human history, technologies with similar characteristics, such as electricity, steam engine, information and communication technologies (ICT), have been classified as General Purpose Technologies (GPT). According to Lipsey, Carlaw, and Bekar (2005), “a GPT is a single generic technology, recognizable as such over its whole lifetime, that initially has much scope for improvement and eventually comes to be widely used, to have many uses, and to have many spillover effects”. Another fundamental category of technology studies is the Invention of a Method of Inventing, or IMI (Griliches 1957). Generally they are research technologies that cause paradigm shifts within the scientific community, increasing research productivity and easing bottlenecks in development and innovation. Understanding the different ways in which technologies’ impact society is vital to guide policymakers to choose the most appropriate policies to foster economic growth and social balance.
The first part of this chapter is dedicated to building a theoretical framework for the technological categorization of General Purpose Technologies, Methods of Invention, and analyzing how they spread in the economy. In the second part of the chapter, I will analyze whether Artificial Intelligence enters in one or more of these categories from a qualitative perspective. Artificial Intelligence is impacting a wide variety of application sectors, affecting productivity, employment, and competition, suggesting that AI should be considered a GPT. Additionally, some scholars have also highlighted that AI can revolutionize the innovation process itself, increasing the number of tools available to researchers and knowledge producers, suggesting it may also be classified as an IMI. This has prompted the scientific community to define a new category that combines the characteristics of GPTs and IMI: General Method of Inventing, or GMI.
Many scholars also affirm that AI can represent a solution to the issue of stagnant productivity. However, the use of AI in the market has both direct and indirect consequences on the market structure, increasing the concentration of capital in a small number of very large firms. Goldfarb and Trefler (2019) affirmed that given the specific characters of the digital economy, AI-powered businesses are changing the way competition takes place, with firms competing for the conquest of new markets. The third part of the chapter focuses on analyzing these phenomenon and presenting some of the externalities caused by the use of AI as a commercial technology.
3.1 A theoretical framework for classifying technologies
3.1.1 General Purpose Technologies
The notion of General Purpose Technologies was first introduced in the economic literature by Bresnahan and Trajtenberg (1995) to identify technologies that have a broad impact on society by promoting both qualitative and quantitative changes in institutional structures, industrial dynamics, allocation of resources, and skill requirements, influencing the macroeconomic dynamics of the international economy. The assumption behind the idea of GPTs is that “major technological changes are the main determinants of cyclical and non-linear patterns in the evolution of the economy” (Cantner and Vannuccini 2012). However, the idea that technology represents an exogenous factor that uniquely influences the direction and rate of change was formulated well before the concept of GPT itself: Schumpeter (1934) noticed that drastic innovations were often followed by long periods of economic growth while Mokyr (1990) uses the term macroinventions to refer to those innovations that were essential complements for the development of a much-increased number of microinventions.
In the economic literature, GPTs are usually identified by the presence of three features:
wide scope for improvement and elaboration: to cause important disruption in the economy, a technology needs to be able to go through a process of rapid technological advance after its first introduction. It is fundamental for the technology to provide the possibility to build knowledge around it cumulatively;
pervasiveness: for a technology to be General Purpose, it must be possible to be used in a wide variety of products and processes, thus making it able to spread across different economic sectors and increase its impact on different industrial compartments;
strong complementarities with existing and new technologies: it must be possible to apply the candidate GPT in combination with existing or future technologies, further increasing the opportunities to develop new technologies. In other words, the productivity of R&D in any downstream sector may increase as a consequence of innovation in the GPT.
Features (1) and (3) are often incorporated under the concept of innovation complementarities, a virtuous cycle of complementary innovation between the GPT sector and the application sectors, as presented by Bresnahan (2010). During a first phase, the GPT technology sparks innovation in the application sectors while at a later stage, the new technologies developed in the application sector generate further innovation in the original GPTs, increasing its technological innovation speed and triggering a positive loop of technological advancement. The overall effect that innovation complementarities have on economic growth largely depends on whether application sectors are capable of implementing the GPT. Suppose the actors in the application sectors manage to do so. In that case, Bresnahan (2010) suggests that the “social increasing returns to scale (SIRS) arise across an entire cluster of technical change in the GPT and technical change in the AS (application sectors) and that GPTs*”may overcome diminished returns because innovation is inherently an increasing returns activity". However, this virtuous cycle of economic growth is not exempt from obstacles and externalities, which will be later discussed.
3.1.1.1 Some words on pervasivesness
Pervasiveness is a GPT quality that describes its “generality of purpose”. From a theoretical perspective, the Schumpeterian model of innovation (Schumpeter 1934), the theory of recombinant growth (Weitzman 1998), combinatorial technology models (Arthur and Polak 2006), and recent studies on technological diffusion (Korzinov and Savin 2016) that consider knowledge as a combination of “pieces” of information that can be connected in one or the other way are helpful to codify such a complex phenomena.
According to Weitzman (1998), new ideas arise from existing ideas in a combinatorial process. He developed the theory of “recombinant innovation”, where old ideas are reconfigured and recombined to produce new ideas. In this framework, the set formed by new ideas is modeled as a subset of the combinatorial set of already existing ideas. The researcher/innovator’s task is to explore the set of old ideas to detect the ones that are more likely to lead to the discovery of new ideas.
Arthur and Polak (2006) operationalize this concept with the help of network science by simulating the process of technological evolution using simple logic circuits. Their work confirms the hypothesis that technological systems can be modeled as a dynamic network of nodes where new nodes are formed as the consequence of the combination between two or more existing nodes. The simulation they performed showed that the formation of new nodes is not only driven by the availability of previous technologies but also by the collection of human needs (provided by the researchers) and the needs of the technologies themselves (the intermediate steps needed in order to reach the research goal). Additionally, some combinations of technologies can become obsolete and practically disappear from the network, paralleling what Schumpeter (1934) defined as “gales of destruction.”
Korzinov and Savin (2016) built on this literature to create a network-based model of technological evolution. They modeled the relation between technologies and final products using a bipartite network of two-layers: final products and technologies (also referred to as intermediates). In the beginning, technologies are not connected. However, to find practical application (and therefore create a final product), they need to become interconnected and form a fully connected component (also referred to as clique).
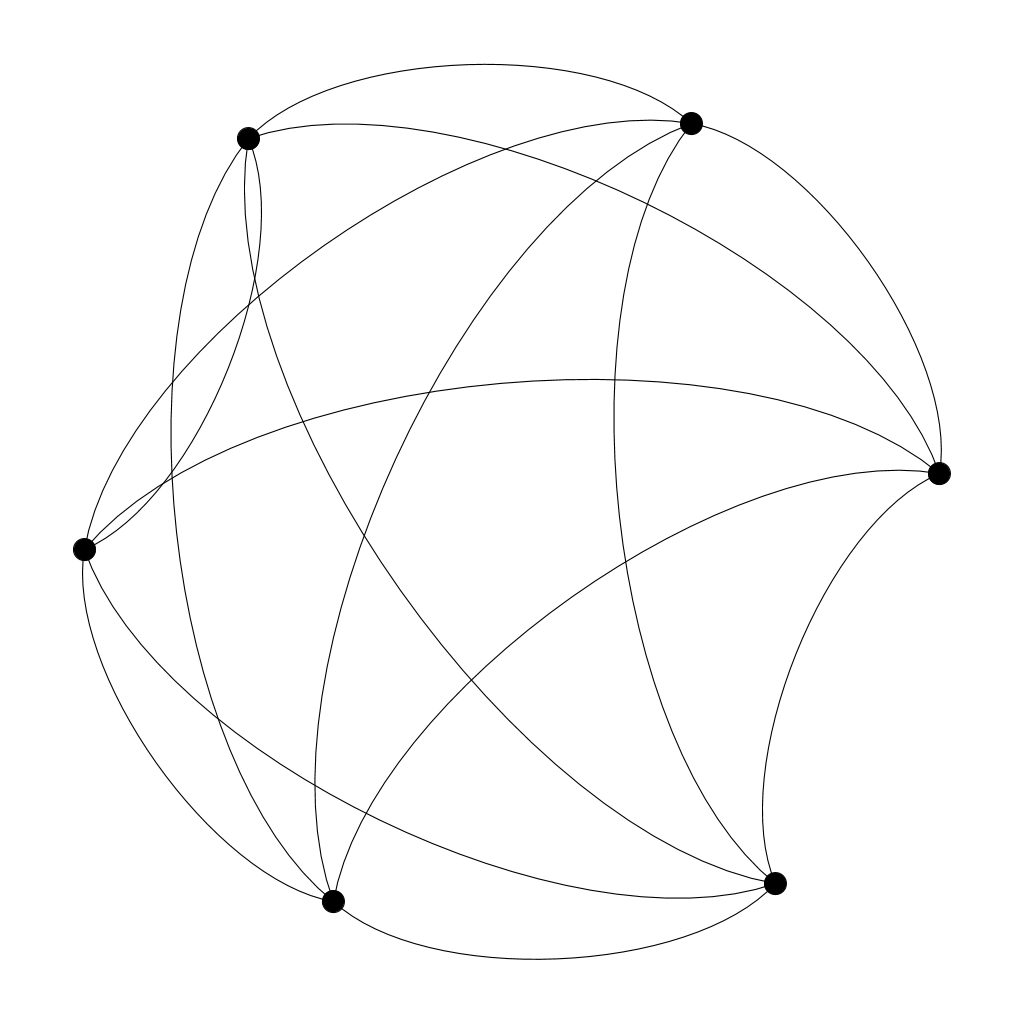
A technological clique composed by six different technologies (nodes) connected with each other
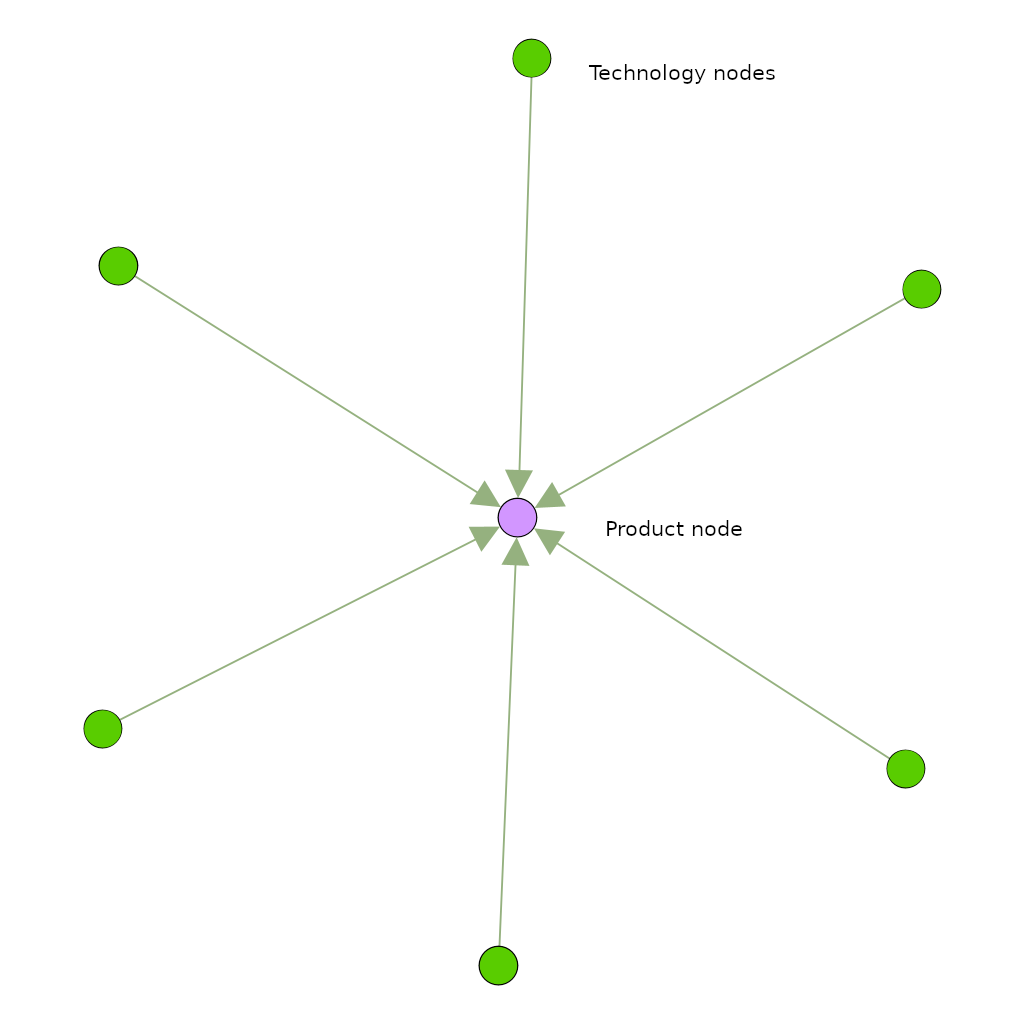
The bipartite representation of the technological clique. The node at the center of the graph represents the product created by the combination of different technologies
In their model, each link between technologies represents the process of knowledge discovery, where “existing technologies are combined with one another in new ways to produce value for a consumer.” Each product that satisfies a specific need can be produced using different technologies (such in the case of substitute goods), and the same combination of technologies may serve to produce different final goods (the complementarity arising from combining different technologies can have more than one application).
By combining all possible technology combinations, one obtains a “potential technology network”. In their model, the potential technology network is not equivalent to a fully connected graph because the structural properties of the technologies limit the network development: not all technologies can be combined, and therefore not all links are possible. In this context, a technology’s pervasiveness may be measured based on the number of links it has with different products. The extent to which a technology may be considered a GPT can then be measured on the degree of its pervasiveness. Alternatively, this pervasiveness can be measured based on its ability to facilitate new bridges between technologies that would not be connected otherwise, or in other words, its brokering capabilities.
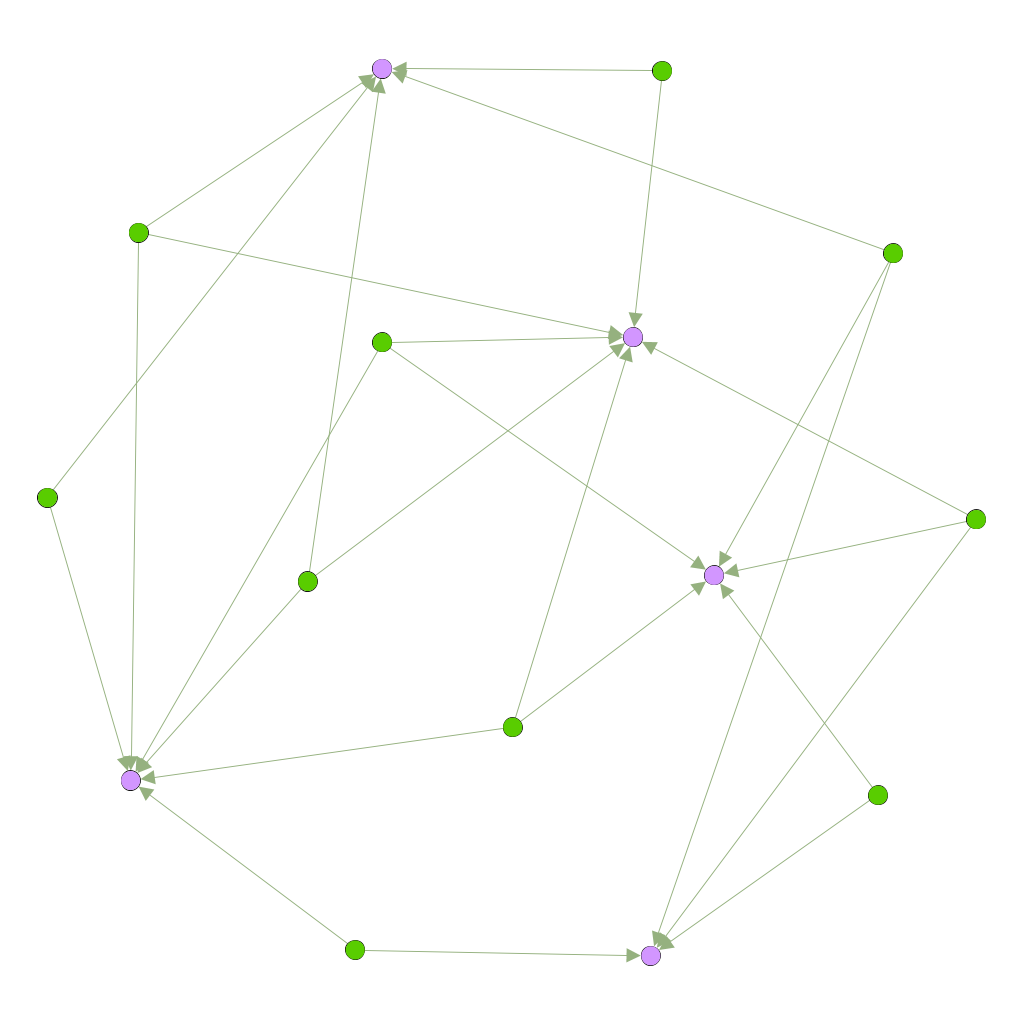
A bipartite technology network. Technologies are represented by yellow dots, while products are represented by blue dots
3.1.1.2 Innovation complementarities
A key feature of GPTs is their ability to spur innovation in the application sectors. The initial assumption is that most technologies are originally introduced as unrefined versions of their best self. As Bresnahan and Trajtenberg (1995) suggested, continuous efforts to innovate a primitive version of the GPT may lead to either a reduction of the ratio between input prices and performance (through learning) or to an increase in the generality of its functional applications. Consequently, the costs of implementation of the GPT for the downstream sector diminish, which may lead to further investments in R&D efforts to profit from the advantages provided by the new technology.
The dynamics of innovation complementarities have been formalized by Bresnahan (2010). Define the technological level of a GPT as \(T_G\), and its rate of change \(T_{G}^{'}\). Apply the same definitions to an application sector \(a\) out of \(A\) application sectors (AS), bringing its respective technological level and rate of change \(T_a\), \(T_{a}^{'}\). The rate of change in the social returns to innovation in \(a\) is a function of the rate of innovation in the GPT, the application sector, and a dummy variable X that stands for other exogenous factors, such as industry and market structure.
\[V_{a}'(T_{G}', T_{a}', X)\]
Note that the social returns for innovation in this function do not take into consideration potential reductions in production costs caused by the technological improvements. Note that the function \(V'_{a}\) has increasing differences in \((T'_{G}, T'_{a})\)24, suggesting that there are social increasing returns if there is coordination in the investments in both the GPT and the application sectors.
Generalizing the effect over time and the entire GPT cluster, the social returns can be expressed as
\[\sum_{a\in A}{\int[V_{a}^{'}(T_{G}^{'}, T_{a}^{'}, X)e^{-rt}] dt}\]
The social returns will be larger if \((T'_{G}, T'_{a})\) are increased together, and will be larger if all of them are increased in a coordinated fashion than if there is not a coordinated increase (Bresnahan 2010).
The success of the virtuous cycle of innovation presented above largely depends on factors such as the specific GPT and application sector’s industry structure, and how private incentives for innovation are distributed between innovative actors. The GPT and applications sectors’ intellectual property regime may increase or reduce the speed of adoption while at the same time having an important influence on the innovation rate. Bresnahan (2010) affirms that, other than these complex dynamics, some basic incentive structures are present in all of them and provides a summary model concerning the returns of innovators. Label \(\lambda_{a}\), \(\lambda_{G}\), \(\lambda_{c}\) the fraction of social value received by the innovators in the application sector, the innovators in the GPT sector, and the consumers, respectively. Consider \(\lambda_{a} + \lambda_{G} + \lambda_{c} \leq 1\), to take into account the deadweight loss caused by systems of appropriation such as patents or trade secrets. Then the returns to investments for an agent operating in an application sector \(a\) is
\[\lambda_{a}\int[V_{a}^{'}(T_{G}^{'}, T_{a}^{'}, X)e^{-rt}] dt\]
*“A higher rate of technical progress in the GPT sector (\(T'_{G}\)), increases both private return to the innovator in* \(a\) and the marginal return to increases in with \(T'_{a}\) . Increasing differences mean that increases in \(T_{G}'\) will increase the incentive for innovators in AS \(a\) to make increases in \(T'_{a}\). Similarly, increases in \(T'_{a}\) will increase the incentive for GPT innovators to make increases in \(T'_{G}\)” (Bresnahan 2010). This virtuous cycle offers the opportunity to overcome diminishing returns into investments in technical change. However, it also creates a trade-off related to the division of the returns between the upstream and downstream sectors. While the upstream innovation makes it possible for the downstream innovation to occur, a higher \(\lambda_{G}\) reduces \(\lambda_{a}\) and the incentive to invest in innovation in the application sector. At the same time, a higher \(\lambda_{a}\), reduces \(\lambda_{G}\). Overall, considering that \(T'_{G}\) depends from the rate of technological innovation in all the sectors \(A\) (\(T'_{A}\)), then the aggregate incentive for investing in GPT innovation may be compensated. From the perspective of a GPT developer (upstream sector), the higher the number of application sectors, the higher the demand, increasing the value of investments in the GPT. Formulating the private returns of investments in the GPT sector:
\[\sum_{a\in A} \left\{ \lambda_{G,a}\int \left[V'_{a} \left(T'_{G} \left( T'_{A} \right), T'_{a}, X \right) e^{-rt} \right] dt \right\}\]
where \(\lambda_{G,a}\) represents the fraction of the social returns obtained by the GPT sector for a specific application sector, out of \(A\) application sectors. In this model, innovators in the application sector pick \(T'_{a}\) to maximize \(\lambda_{a}V'_a\). If instead they aimed to maximize the rate of innovation at all levels, they would pick \(T'_{a}\) to maximize the effects on the whole production function \(\left(\lambda_{a} + \lambda_{G}\right)V'_{a}\).
Other than this “vertical” externality, Bresnahan (2010) and Bresnahan and Trajtenberg (1995) affirm there is also a second, horizontal externality, that arises from the interaction between multiple application sectors. Any innovation or increase in investments in the GPT makes them better off by raising the GPT quality. The same is true for R&D investments in any other application sector because innovations in any of the application sector increase the rate of innovation of the GPT sector, which, in turn, increases the rate of innovation in the application sectors, including those that invested in the first place, as an effect of the positive cycle of innovation complementarities. Simultaneously, innovators in the application sectors act in such a way to maximize the returns for their investments in innovation, which largely depends on the degree of investments in other sectors. However, if every application sector follows the same strategy this behavior can result in a standoff, where application sectors invest the minimum necessary amount, slowing the pace of innovation. This issue can be partially solved by improving coordination, but that can prove complicated because the large number and variety of application sectors increase information asymmetries. So, in the presence of innovation complementaries between the GPT and application sector, the lack of incentives in one sector can create an indirect externality that results in a system-wide reduction in the innovative investment itself (Bresnahan and Trajtenberg 1995). In the past, coordination was partially achieved because of the existence of predictable large demanders that had other incentives to sustain conspicuous fixed costs such as the strategic importance of the industry (in the case of public procurement) or to boost their technological dynamism (in the case of the private sector).
3.1.1.3 Time implementation
The concept of a time delay between the moment a technology is invented and when it enters into the economy is well established in the innovation literature. Considering that the innovation dynamics of a GPT are marked by the complex dynamics described above, it is not surprising that GPTs implementation lag is generally larger than average. As Bresnahan (2010) noted, “the very idea of a GPT draws a distinction between raw technical progress (GPT invention) and the further innovation needed to create value-in-use (application sector invention.)”. In the economics of innovation, implementation is generally modeled as an S-shaped diffusion curve (slow at the beginning, followed by a period of rapid growth, and then reaching an innovation plateau) that may depend on a variety of factors, such as supply or demand constraints, adjustment costs related to the need to substitute previous technologies, or information asymmetries.
Helpman and Trajtenberg (1994) suggest that GPTs spread in two phases: one in which the resources are diverted to the development of complementary inputs needed to take advantage of the new GPT, where productivity and stage output growth are negative, and a second phase, after the complementary inputs are already developed, where it is worthwhile to switch manufacturing with the new, more productive, GPT, that is characterized by an enormous increase in stage output, rising wages, and profit. In a later paper (Helpman and Trajtenberg 1996), they expanded the model to consider the fact that multiple application sectors are operating contemporaneously in the real world. They determined that, while the initial stages of the spread of the new GPT are characterized by intermittent growth25, after a “critical mass” of application sectors have made the necessary complementary investments, we assist to a surge in growth, together with a rise in wages and profit. Their models show that there may be various factors affecting this diffusion process and that shape the rate and the trajectory of the transition towards a new GPT, such as the rate of adoption, the technological distance of the new GPT from the previous one (such as the transition between steam power and electricity), the propensity to invest in R&D, the order of adoption of the various application sectors, and other exogenous factors. Moreover, it is essential to note that different adoption orders in the application sectors may trigger multiple second phases (those characterized by rising productivity).
3.1.2 Methods of Inventing
Research technologies are essential tools for innovation. They provide new ways to produce knowledge, opening new scientific fields and expanding the knowledge frontier of what it is possible to discover. Griliches (1957) was the first economist that brought to attention the critical role that the development of new research tools has on the innovation process. He did so during a study on hybrid corn, founding the technological category of IMI. However, even if he formalized this concept, the definition that he provides: “It was not a single invention immediately adaptable everywhere”, together with the econometric evidence that shows multiple waves of innovation, appears to blur the differences between GPTs and IMIs. Some scholars, such as Darby and Zucker (2003), misapplied Griliches’ breakthrough concept to the field of nanotechnology, limiting it to be an invention that provides a business opportunity across a variety of ranges. On the other hand, [Cockburn, Henderson, and Stern (2018)] suggest a different idea of IMI, where they are closer to innovations in research tools or innovation in processes. This seems to be more in line with the initial intuition of Griliches since the value of the invention of hybrid corn was not in the hybrid corn itself rather than in the invention of a “widely applicable method for breeding many different new varieties” (Cockburn, Henderson, and Stern 2018). Hentschel (2015) analyzed how some scientific instrumentation shifts from the specific context in which it is developed, with a particular use, towards a more general use, mentioning the invention of optical lenses. Putting two lenses in line allowed for the first time to observe things that were not possible to discern at the naked eye and that completely changed the scientific paradigms of entire research fields, such as medicine, astronomy, and chemistry, and allowed the development of new research fields. This reduced the gap between theory (what was thought to be) and the empirical observation of real phenomena (what it is possible to experience), altering the preindustrial scientific community’s scenario. “much of the seventeenth-century scientific revolution was made possible by better instruments and tools” (Mokyr 2018), which, in turn, were the prerequisites for the explosion of productivity of the first industrial revolution. Another famous example of IMI is the much-debated Oncomouse patent, whose applicants aimed to expand the patent scope to the method for genetically engineering. This sparked outrage in many researchers, which refused to pay royalties for a fundamental and general method of biotechnology.
Differently from the concept of GPTs, the applications of an IMI are limited to a single (or a small number of) application sector, such as agriculture (in the case of hybrid corn) or biotechnology (in the case of the Oncomouse). IMIs can reduce the cost of specific innovation activities while shaping the development of new “innovation playbooks” (Cockburn, Henderson, and Stern 2018). They expand the number of tools available to the researcher, thus extending the addressable research question’s horizon and enhancing the rate of innovation and discovery.
Recalling the theory of recombinant innovation by Weitzman (1998) and the network-based technology model developed by Korzinov and Savin (2016), new IMIs act as “facilitators” in finding useful connections between different pieces of knowledge. In network science terms, nodes in the network represent ideas, and edges represent their combination. The introduction of a new IMI increases the likelihood of creating an edge between previously interconnected nodes and of reinforcing existing ones. While traditional indicators of innovation such as patents and scientific papers seem to be increasing at increasing rates, recent empirical evidence indicates that research productivity has been decreasing and that new ideas are getting harder to find (Bloom et al. 2020). This seems to confirm what Weitzman (1998) proposed in his original paper, stating that the limit for the generation of new ideas (innovation) is marked not by the potential to generate them but rather by the ability of researchers to process them and evaluate their validity. The number of possible combinations of ideas increases at a much higher rate than the research community’s ability to evaluate them, but the cognitive abilities of human beings can only process a limited amount of information. The knowledge landscape is not only becoming vaster and vaster but also more fragmented. The expansion of science leads to increased specialization, the flourishing of sub-disciplines, and researchers can focus on fewer and fewer topics (Jones 2009). Thus, in the absence of improvements in research methodologies, the pace of innovation is bound to decrease. Improvements in IMIs may partially solve this issue.
While the impact of new research tools on innovation is a well-established concept in innovation studies, currently there is no econometric model that tries to explain its complex dynamics. However, formalization is fundamental to understand how the evolution of research tools influences technological advancements, especially now that the literature has started noticing several technologies that possess features typical of GPTs and IMIs. Drafting a formal definition for IMIs:
they expand the knowledge frontier of science by increasing the set of tools available to researchers;
they reduce the distance between recombinable ideas, increasing the rate of scientific advancement;
they have genericity of use: they can be applied for inventing different products in a limited number of application sectors.
At first glance, it may seem that the genericity of IMIs can be assimilated to the pervasiveness of GPTs. However, while the former involves the possibility to use a method or a technique as an intermediate step within an application sector for the development of new methods and technologies, the latter is about the ability of a technology to spread across different application sectors of the economy as a final product.
3.1.3 General Methods of Invention
In rare cases, some technologies present features of both GPTs and IMIs, leading scholar to come up with a new technological category: General Method of Invention. “A GMI is a (I)MI that is applicable across many domains. Similar to GPTs, development of the GMI and complementary developments in application domains are mutually reinforcing” (Bianchini, Müller, and Pelletier 2020). Given that such technologies are extremely scarce, there is not a shared terminology yet, and they have been called using different nomenclatures, such as General Method of Invention, General-Purpose Invention in the Method of Inventing or General Meta-technology (Bianchini, Müller, and Pelletier 2020; Cockburn, Henderson, and Stern 2018; Agrawal, McHale, and Oettl 2018). For this dissertation, I retain that the term suggested by Bianchini, Müller, and Pelletier (2020) is particularly descriptive, thus I will refer to this class as General Method of Invention (GMI).
As one can imagine, GMIs have an immense potential for exponentially increasing the innovation rate. At present, the innovation literature agrees only on one of such technologies: digital computing. Computing technologies had an enormous impact across economies and societies, of which we are still experiencing its full potential. Recently, AI is increasingly regarded as a GMI candidate. Unfortunately, the absence of previous examples of such technologies and the complex nature of how they affect the economy prevents scholars and researchers from identifying precise characterizations.
3.2 Is AI a GPT?
The theoretical framework presented in the previous section is crucial to understand how AI technologies will impact the economy. After the commercial success of the new generation of AI technologies, several papers have suggested that AI should be categorized as a GMI, since it is possible to identify features of both GPTs and IMIs. Recalling the definition provided in section 1.1.1, a technology is considered a GPT if it has a broad scope for improvement and elaboration, if it can be applied in a large number of sectors of the economy, and if it has strong complementarities with existing and new technologies. From a qualitative perspective, AI fulfills these the GPT requirements:
3.2.0.0.1 Rapid growth
AI algorithms can improve themselves over time through learning, further enhancing their potential applications. Pratt (2015) identified as one of the core capabilities of AI its ability to share knowledge and experiences almost instantaneously, provided an internet connection. In this way, once a machine learns a new skill, that piece of knowledge can be shared with all the other devices in the network, thus increasing the data available and improving performance, further extending the possible fields of application.
3.2.0.0.2 Pervasiveness
As it was mentioned in chapter one, modern AI technologies are essentially prediction technologies, which are a crucial enabler for improving decision-making, which is present in any sector to different degrees. Decision-making may involve choosing where to store materials in a storage unit, whether or not to operate a patient suspected of having a tumor, or selecting the best financial assets for a long-term investment. AI technologies may improve speed and precision, desirable performance indicators in a variety of different areas. Implementations of AI technologies already surround us: machine translation has spread widely; search engines use AI to select the best results according to our query; it is possible to use speech-to-text to control devices remotely; we increasingly rely on navigation apps to find the best route in unknown environments. AI is impacting several sectors of the economy, and the number of its applications is increasing steadily.
3.2.0.0.3 Strong complementarities with existing sectors
The introduction of AI in various application sectors has the potential to spur complementary innovation. New methods must be found to increase productivity, and new challenges need to be faced. Solutions found in the downstream sector can later be applied in the upstream sector, starting a virtuous cycle of innovation in algorithms and data management.
3.2.1 The role of AI in the research process
Artificial Intelligence applications are not limited to commercial settings. Since their early developments, AI technologies found most of their applications in academia. Most quantitative research regards finding patterns and causal relationships, and ML systems are particularly efficient when dealing with high-dimensional data. The development, optimization, and application of multi-layer neural networks to these sets of data allowed researchers to expand the set of research questions that can be feasibly addressed, thus changing the way research is conducted (Cockburn, Henderson, and Stern 2018). Agrawal, McHale, and Oettl (2018) distinguished two different uses of AI in research: search and discovery. The search stage concerns finding relevant information for the researcher, drawing from different fields. In other words, AI tools involved in the search process aim to optimize the initial stage of the researcher’s work, facilitating the process of identification of the critical factors to take into account when investigating a phenomenon. In the discovery stage, AI tools are more focused on predicting which “combinations of existing knowledge that will yield valuable new knowledge across a large number of domains” (Agrawal, McHale, and Oettl 2018). In the following paragraphs, both of these aspects are exposed in detail.
3.2.1.1 Limited resources, unlimited knowledge
According to Bornmann and Mutz (2015), scientific papers are subjected to average growth of 8 to 9 % per year. Considering the increasing investments in research activities, the upward trend in the number of PhD graduates, and the tendency towards knowledge fragmentation, it is doubtful that individual researchers can deal with the constantly increasing knowledge at their disposal. A temporary solution was found by expanding the size of research teams, but it is not sustainable in the long-term. In this context, AI assistants can prove to be a valuable asset. Various AI-based tools have been developed to help researchers navigate significant amounts of unstructured knowledge, typically in the form of search engines or knowledge graphs.
Perhaps Google Scholar is the most known AI-based tool for identifying papers and studies given a keyword-based input, but also the less sophisticated one. It is generally used to navigate papers and books, find cited/citing papers, and obtain bibliographic information. In recent times, Semantic Scholar, another search-engine developed by the Allen Institute for Artificial Intelligence in Seattle, has gained popularity. Whereas the ranking function of Google Scholar is mostly based on citation indices, the approach of Semantic Scholar relies on a semantic analysis of papers’ titles and abstracts, thus making it possible to obtain more accurate results and optimizing it for scientific discovery, allowing for a more relevant and efficient view of the literature of choice. It was initially used for computer science, geoscience, and neuroscience, but it has been progressively extended to other academic areas. It provides additional functionalities, such as the construction of personalized Research Feeds based on a small group of papers chosen by the users, or the TL-DR function (Too Long, Didn’t Read), that summarizes the abstract of scientific articles in maximum two lines. Used in the context of biomedical research, Meta is a machine-learning web platform that aims “to map biomedical research in real-time”. By analyzing and connecting millions of scientific outputs, it aims to provide researchers with a comprehensive view of how biomedicine sub-fields are evolving. Substantially different from the other mentioned tools, SourceData is a data discovery tool promoted by the European Molecular Biology Organization (EMBO) that explicitly targets biomedical scientists. It tries to address the issue of biomedical data locked away in published scientific illustrations that are not indexed by traditional search tools. Starting from the metadata that describe scientific figures, SourceData converts them in a standardized format that allows for the linkage of data originated by different experimental papers. In this way, it aims to reduce the inefficiencies caused by the lack of structured communication within the biomedical scientific community.
3.2.1.2 AI as a research technology
In the second stage of the research process, discovery, Artificial Intelligence technologies can effectively be used to speed up experimental validation and/or dealing with high dimensional data.
Recalling the definition of the knowledge space provided by the theory of recombinant innovation (Weitzman 1998), where the successful combination of ideas originates new ideas, improvements in prediction technologies (such as AI) can result in fewer resources wasted during the research process. In any research effort, prediction26 is used to map all the possible combinations of ideas to prioritize those that are more likely to yield new knowledge. Assuming that inputs are the already existing ideas and data over past combinations, the prediction function provides as output the probability for each possible combination to lead towards a successful new idea. These probabilities can be ranked to promote the prioritization of the combinations that are more likely to lead to a successful idea, helping scientists to choose the right path. Consequently, every improvement in prediction technology represents a potential source of increased productivity in the research output.
Compared to other prediction technologies, such as parametric modeling, AI has the advantage of being particularly suited for discovering non-linear relationships in high-dimensional data. While parametric modeling techniques are constrained by the need to extract features (explanatory variables) by hand before the statistical analysis, algorithmic modeling considers the relations between input data as complex and unknown. In parametric modeling, the analyst specifies the dependent variable, the predictor variables, the functional form of the relationship between them, and the stochastic form of the disturbance term. Using theory, the researcher explicitly formulates the association of real-world data to the variables. While this approach has the advantage of allowing total explainability, when it is used to analyze more complex phenomena, the human brain cannot manage so many variables and information, undermining the model validity.
Instead, DL-based prediction aims to find the function \(F(x)\) that from \(x\) predicts the response \(y\). The validity of the model is measured by the accuracy through which the algorithm predicts the correct output. Advances in AI architecture allowed for substantial improvement in high-dimensional spaces with complex non-linear interactions, giving AI a comparative advantage over parametric modeling in analyzing large amounts of real-world data (Agrawal, McHale, and Oettl 2019). Theory is present, but it enters the research pipeline in a different stage. Rather than being used to build a predictive model (as in parametric modeling or educated guesses), it is used during previous and later phases such as data gathering, experimental design, and result interpretation. Recently, these steps have started a process of automation under the supervision of the researcher. Representation learning automatically extracts what the algorithm identifies as the most relevant features. Robotic laboratories are used to design and perform experiments. Theory is used to understand why AI chooses them, to rule out algorithmic confusion and biases and build new conceptual frameworks.
When the challenges presented are combinatorial, the discovery process has the potential to be entirely automated. Examples of such research questions are “how do I combine molecules to create a new material with these characteristics?” or “how do I build a drug that has these specific effects?”. One of the most promising applications of such closed-loop platforms is material discovery, where the combinatorial space of the molecules can be analyzed using Deep Learning to predict the structure of new materials and their properties. This process is known as inverse design (Sanchez-Lengeling and Aspuru-Guzik 2018). An initial library of molecules selected by the researcher is filtered based on focused targets such as ease of synthesis, solubility, toxicity, and stability. Closing the loop would require integrating these simulations in a robotic laboratory able to conduct experiments. According to Aspuru-Guzik and Persson (2018), current research related to closed-loop systems for material discovery is focusing on improving “AI-based predictions of new materials and their properties, autonomous robotic systems for synthesis and experimental data collection, data analysis techniques such as feature extraction, AI-based classification and regression of results, and decision modules to drive optimal experimental design for subsequent experimental iterations”. Currently, the bottlenecks of the process are represented by experimental design, where human intuition still plays a fundamental role, and the lack of data on “failed” experiments, crucial to teach the AI how to build an integrated research pipeline. Machine learning methods such as deep learning are indeed a promising tool for discovery, especially where the complexity of the phenomena makes it difficult for the researcher to adopt more traditional methods. However, even in these limited cases, the analysis they provide still needs to be subjected to experimental validation and thorough examination by human researchers.27
3.3 AI in the market
The extent to which Artificial Intelligence can be considered a GPT or a GMI has significant consequences for economic growth and innovation but these technologies are already likely disrupt the economy in unexpected ways. In recent times, some scholars started investigating the effects of the deployment of AI systems on market structure, competition, and the consumers’ decision-making processes.
3.3.1 AI and market structure
Without the introduction of specific legislation, AI technologies are likely to enhance current disruption provoked by the expansion of the scope of the digital market. Coupled with an enormous decrease in labor per customer ratio, the digital economy is already marked by extreme network externalities and strong economies of scale and scope that may result in anti-competitive behavior. In a report on competition Crémer, Montjoye, and Schweitzer (2019) explored the mechanisms of the digital economy in detail, identifying three key features of the digital market:
extreme returns to scale: after having surpassed a threshold, digital products have a marginal cost of consumption that is close to zero. This brings a significant competitive advantage for an already established business. Moreover, data present increasing returns to scale. While in terms of performance, data exhibits decreasing marginal returns (accuracy improves at a decreasing rate according to the quality and quantity of data supplied during training), a firm may experience a comparative advantage in providing a service that is just marginally better than its competitors. A slightly better prediction leads to a better service, which in turn leads to a higher share in the market Goldfarb and Trefler (2019);
network externalities: in many cases, the attractiveness of a product or service relies on the number of users that already use it, such as in the case of messaging services or social networks. After a business has conquered market shares, it may be challenging for newcomers to convince users to migrate to its products, even when their products are cheaper or more innovative. Additionally, the incumbent may be actively promoting its own complementary service;
data advantage: first-movers have no incentive to promote easy transfers of users’ data towards competitors, and the current IPRs framework on database rights leave space for ownership claims regarding compilation of users’ data, thus giving grounds for the systematic obstruction of the transfer of users’ data and information towards competitors. Additionally, users’ data can be used to develop new innovative products, thus providing them with a competitive advantage in innovation (Goldfarb and Trefler 2019).
These mechanisms are leading towards increased concentration of the profits generated in the digital economy in a few dominant platforms that have strong incentives to reinforce their dominant position (Crémer, Montjoye, and Schweitzer 2019). Bloom et al. (2014) suggest that widespread use of AI technologies may speed up the concentration process.
Additional concerns related to the widespread use of AI technologies are related to data ownership. Since data are an essential input for all AI-based technologies, firms that own large datasets may create barriers to entry, further increasing economic externalities. Goldfarb and Trefler (2019) suggest that “competition in the market is substituted by competition for the market”. This is because, in AI-powered business, both the expenses related to personnel and data acquisition can be optimized to reach a variety of objectives such as the development of new products, optimizing the total costs. The lack of exploitation of already gathered data would result in firm-level inefficiencies because businesses would not have extracted their full value. Then the firm has two choices: either sell the data or invest in R&D to expand the pool of goods that it puts into the market. However, privacy legislation may impose severe restrictions on buying and selling data, thus disincentivizing the creation of a data market. This mechanism increases the firm’s incentive to find new ways to produce economic value from the unused data in its possession through innovation and expansion in new markets. However, while this mechanism may have a positive effect on innovation (Nuccio and Guerzoni 2019), the absence of a data market impedes other actors from enjoying the innovation benefits derived from data repurposing and thus favors incumbents over new entrants. Multipurpose firms such as Google, Microsoft, Apple, Facebook, and Amazon can only allocate a certain amount of resources to R&D compared to data repurposing possibilities. The exclusive ownership by a firm of a particular set of data can potentially erect data-driven entry barriers to markets that have not come into existence yet, thus impairing the speed of innovation. Additionally, this provides corporations possessing large datasets with an extreme prescriptive power over the direction of innovation (Cockburn, Henderson, and Stern 2018). On the other hand, forcing the incumbent to share data could diminish the incumbent incentive to invest in data creation, obtaining a reduction in the pace of innovation. An alternative proposition made by Korinek and Ding Xuan (2018) points out that one way to reduce inequality and anti-competitive behavior may be to reduce the rents earned by innovators derived from IPRs, specifically patents, but this may result in a lack of technology disclosure, thus slowing down innovation and reducing transparency over the treatment of users’ data.
3.3.2 Some market externalities of Artificial Intelligence
The benefits brought by AI technologies are affecting both firms and consumers. Notably, there are cost-reductions in search, replication, transportation, tracking, and verification (Goldfarb and Tucker 2017). Algorithms can improve consumers’ decision-making by organizing information based on price information and other criteria, such as product quality and consumers’ preference. However, the spread of AI technologies can also provoke market failures, such as behavioral biases and cognitive limits. Decreased search costs do not necessarily favor consumers. As argued by Goldfarb and Tucker (2017), service providers and companies can elaborate strategies to manipulate the search process to trick consumers and increase profits. While AI technologies positively affects the supply side of the market by reducing production costs, increasing the quality of existing products, and improving resource allocation, it is also used to provide optimal commercial strategies to sellers that can use Recurrent Neural Networks to maximize their profits. While a complete review of these mechanisms is outside of the scope of this dissertation28, I will briefly introduce how AI may impact the market dynamics through the use of dynamic pricing.
3.3.2.1 Dynamic pricing
Dynamic pricing technologies optimize and adjust prices based on factors such as stock availability, capacity constraints, competitors’ prices, or demand fluctuations. On the bright side, this allows the market to constantly be in equilibrium, thus preventing the mismatch between demand and excess of supply. On the other hand, this puts sellers that do not use these technologies (for various reasons, such as investment cost, low innovation capabilities, or others) at a disadvantage, and makes consumers’ decision process more difficult, since they are forced to buy in an environment characterized by constant price fluctuations. While for some aspects, this has a positive effect since it puts companies under the pressure to innovate (Cockburn, Henderson, and Stern 2018), there is a concrete risk of algorithmic collusion and thus a reduction of the consumers’ surplus.
Moreover, for the first time in economic history, dynamic pricing provides an opportunity to systematically apply first-degree price discrimination29. Through extreme personalization obtained by gathering data on users’ online behavior, platforms can often deduce the consumer’s reservation price and differentiate the price (Milgrom and Tadelis 2018). Gautier, Ittoo, and Van Cleynenbreugel (2020) presented some examples of such practices30, even if it seems that, for the moment, they are not the industry standard. They suggest that this may be attributed to different factors, such as technical barriers and consumers’ perception31.
The use of dynamic pricing also creates issues related to algorithmic collusion. Several experiments were drawn on sample market structures to understand how the interaction of multiple automatic pricing systems may take place. Last-generation algorithmic pricing is based on ML techniques, such as Recurrent Neural Networks, in which the software learns the optimal strategy by trial and error without requiring any specification related to the economic model. Klein (2019) showed that well-established algorithms learn autonomously to set supra-competitive prices in a sequential game. Calvano et al. (2020) in another experiment show that when multiple dynamic pricing algorithms are left to interact with simultaneous moves, they learn to play sophisticated collusive strategies, difficult to detect. Unlike human-based collusion, AI-based collusive strategies are “robust to perturbations of cost or demand, number of players, asymmetries and forms of uncertainty”. This is particularly problematic for antitrust authorities since algorithmic collusion has no trace of concerted action (automatic pricing systems do not communicate with one another), thus escaping the current regulatory framework. Additionally, liability determination is problematic, as in all situation where AI systems actively take decisions autonomously.
3.4 Conclusions
This chapter touched on many arguments related to the effects of the widespread diffusion of Artificial Intelligence technologies in the economy. First, it provided a theoretical background for the classification of technologies based on their impact on innovation. We have seen that General Purpose Technologies may bring an increase in productivity and, as Bresnahan and Trajtenberg (1995) suggested, they can become “engines of growth” creating a virtuous cycle of innovation. “Inventions of a Method of Inventing” identify research tools as one of the key elements for increases in the rate of innovation. Combining these two categorizations, many scholars have argued in favor of the development of the concept of General Method of Inventing, which include the technologies with characteristics of both IMIs and GPTs. Second, some qualitative evidence was provided regarding how Artificial Intelligence technologies should be included in any of these categories. The evidence suggests that AI can be both used in various commercial settings and as a research technology, indicating that it is a potential GMI candidate. On the other hand, given the rarity of these technologies, their economic effects are challenging to forecast, even if they likely increase the rate of innovation and technological change, increasing the productivity of both research and industrial output. Finally, a point was made on the effects of AI in market structure and some of the externalities of the widespread use of AI as a market tool. In the absence of appropriate regulation, AI technologies will likely increase the speed of concentration of market power in a few players that do not compete with each other in markets but that primarily compete for the conquest of new markets, constantly expanding the scope of their economic activities. These conglomerates are in an advantageous position when compared with new entrants because of the repurposing capabilities of data. Moreover, when AI technologies are introduced in market transactions, they indeed bring some benefits to both consumers and producers, but they can also create negative externalities caused by their widespread use, such as dynamic pricing, first-degree price discrimination and algorithmic collusion.
References
Abrardi, Laura, Carlo Cambini, and Laura Rondi. 2019. “The Economics of Artificial Intelligence: A Survey.” Vol. 58. Robert Schuman Centre for Advanced Studies Research Paper No. RSCAS.
Agrawal, Ajay, John McHale, and Alex Oettl. 2018. “Finding Needles in Haystacks: Artificial Intelligence and Recombinant Growth.” National Bureau of Economic Research.
Agrawal, Ajay, John McHale, and Alexander Oettl. 2019. “Artificial Intelligence, Scientific Discovery, and Commercial Innovation.”
Arthur, Brian, and Wolfgang Polak. 2006. “The Evolution of Technology Within a Simple Computer Model.” Complex. 11: 23–31.
Aspuru-Guzik, Alán, and Kristin Persson. 2018. “Materials Acceleration Platform: Accelerating Advanced Energy Materials Discovery by Integrating High-Throughput Methods and Artificial Intelligence.” Mission Innovation. Canadian Institute for Advanced Research.
Bianchini, Stefano, Moritz Müller, and Pierre Pelletier. 2020. “Deep Learning in Science.”
Bloom, Nicholas, Luis Garicano, Raffaella Sadun, and John Van Reenen. 2014. “The Distinct Effects of Information Technology and Communication Technology on Firm Organization.” Management Science 60 (12): 2859–85.
Bloom, Nicholas, Charles Jones, John Van Reenen, and Michael Webb. 2020. “Are Ideas Getting Harder to Find.” The American Economic Review 110: 1104–44.
Bornmann, Lutz, and Rüdiger Mutz. 2015. “Growth Rates of Modern Science: A Bibliometric Analysis Based on the Number of Publications and Cited References.” Journal of the Association for Information Science and Technology 66 (11): 2215–22.
Bresnahan, Timothy. 2010. “General Purpose Technologies.” In Handbook of the Economics of Innovation, 761–91. Elsevier.
Bresnahan, Timothy, and Manuel Trajtenberg. 1995. “General Purpose Technologies ‘Engines of Growth’?” Journal of Econometrics 65 (1): 83–108.
Calvano, Emilio, Giacomo Calzolari, Vincenzo Denicolò, and Sergio Pastorello. 2020. “Artificial Intelligence, Algorithmic Pricing and Collusion.” American Economic Review 110 (10): 3267–97.
Cantner, Uwe, and Simone Vannuccini. 2012. “A New View of General Purpose Technologies.” Jena Economic Research Papers.
Cavallo, Alberto. 2018. “More Amazon Effects: Online Competition and Pricing Behaviors.” National Bureau of Economic Research.
Cockburn, Iain, Rebecca Henderson, and Scott Stern. 2018. “The Impact of Artificial Intelligence on Innovation.” National bureau of economic research.
Crémer, Jacques, Yves-Alexandre de Montjoye, and Heike Schweitzer. 2019. “Competition Policy for the Digital Era.” European Commission.
Darby, Michael, and Lynn Zucker. 2003. “Grilichesian Breakthroughs: Inventions of Methods of Inventing and Firm Entry in Nanotechnology.” Working Paper 9825. National Bureau of Economic Research. \url{http://www.nber.org/papers/w9825}.
Gautier, Axel, Ashwin Ittoo, and Pieter Van Cleynenbreugel. 2020. “AI Algorithms, Price Discrimination and Collusion: A Technological, Economic and Legal Perspective.” European Journal of Law and Economics 50: 405–35.
Goldfarb, Avi, and Daniel Trefler. 2019. “Artificial Intelligence and International Trade.” In The Economics of Artificial Intelligence: An Agenda, 463–92. National Bureau of Economic Re.
Goldfarb, Avi, and Catherine Tucker. 2017. “Digital Economics.” Microeconomics: Search; Learning; Information Costs & Specific Knowledge; Expectation & Speculation eJournal 57 (1): 3–43.
Griliches, Zvi. 1957. “Hybrid Corn: An Exploration in the Economics of Technological Change.” Econometrica 25 (4): 501–22.
Helpman, Elhanan, and Manuel Trajtenberg. 1994. “A Time to Sow and a Time to Reap: Growth Based on General Purpose Technologies.” National Bureau of Economic Research.
Helpman, Elhanan, and Manuel Trajtenberg. 1996. “Diffusion of General Purpose Technologies.” Working Paper 5773. National Bureau of Economic Research.
Hentschel, Klaus. 2015. “A Periodization of Research Technologies and of the Emergency of Genericity.” Studies in History and Philosophy of Modern Physics 52: 223–33.
Jones, Benjamin. 2009. “The Burden of Knowledge and the ‘Death of the Renaissance Man’: Is Innovation Getting Harder?” The Review of Economic Studies 76 (1): 283–317.
Klein, Timo. 2019. “Autonomous Algorithmic Collusion: Q-Learning Under Sequential Pricing.” Amsterdam Law School Research Paper.
Korinek, Anton, and Ng Ding Xuan. 2018. “Digitization and the Macroeconomics of Superstars.”
Korzinov, Vladimir, and Ivan Savin. 2016. “Pervasive Enough? General Purpose Technologies as an Emergent Property.” Working Paper Series in Economics. Karlsruhe Institute of Technology (KIT), Department of Economics; Management.
Lipsey, Richard, Kenneth Carlaw, and Clifford Bekar. 2005. Economic Transformations: General Purpose Technologies and Long-Term Economic Growth. OUP Oxford.
Milgrom, Paul, and Steven Tadelis. 2018. “How Artificial Intelligence and Machine Learning Can Impact Market Design.” Working Paper 24282. National Bureau of Economic Research.
Mokyr, Joel. 1990. “Punctuated Equilibria and Technological Progress.” The American Economic Review 80: 350–54.
Mokyr, Joel. 2018. “The Past and the Future of Innovation: Some Lessons from Economic History.” Explorations in Economic History 69: 13–26.
Nuccio, Massimiliano, and Marco Guerzoni. 2019. “Big Data: Hell or Heaven? Digital Platforms and Market Power in the Data-Driven Economy.” Competition & Change 23 (3): 312–28.
Pratt, Gill. 2015. “Is a Cambrian Explosion Coming for Robotics?” Journal of Economic Perspectives 29 (3): 51–60.
Sanchez-Lengeling, Benjamin, and Alán Aspuru-Guzik. 2018. “Inverse Molecular Design Using Machine Learning: Generative Models for Matter Engineering.” Science 361 (6400): 360–65.
Schumpeter, Joseph. 1934. The Theory of Economic Development: An Inquiry into Profits, Capital, Credit, Interest, and the Business Cycle. Transaction Publishers.
Townley, Christopher, Eric Morrison, and Karen Yeung. 2017. “Big Data and Personalized Price Discrimination in Eu Competition Law.” Yearbook of European Law 36: 683–748.
Weitzman, Martin. 1998. “Recombinant Growth.” The Quarterly Journal of Economics 113 (2): 331–60.
Name \(T'_{G} = g\) and \(T'_{a} = a\), thus \(V'_{a}(g,a,X)\). Suppose \(g \leq g'\), and \(a \leq a'\) for all \(X\). Then \(\Delta_{g,g'}V'_{a}(a) = V'_{a}(g',a,X) - V'_{a}(g,a,X)\), making the function \(\Delta_{g,g'}V'_{a}\) non-decreasing for all \(a \leq a'\). Thus \(\Delta_{g,g'}V'_{a}(a) \leq \Delta_{g,g'}V'_{a}(a')\), that can be shaped as \(V'_{a}(g',a,X) - V'_{a}(g,a,X) \leq V'_{a}(g',a',X) - V'_{a}(g,a',X)\)↩︎
Since the complementary investments are used only by a few early adopters↩︎
In its different forms, such as educated guesses, theoretical models, statistical parametric modeling, or others↩︎
In the appendix Autonomous Discovery Systems is discussed the current debate on Autonomous Discovery Systems↩︎
See Abrardi, Cambini, and Rondi (2019) for a more complete review.↩︎
First degree price discrimination occurs when prices at which goods and services are sold are equal to consumers’ reservation price↩︎
Such as in the case of Amazon’s scandals regarding price discrimination over DVDs or mahjong tiles. See Cavallo (2018) and Townley, Morrison, and Yeung (2017) for reference.↩︎
Since first-degree discrimination is widely considered an exploitative practice, when aware of these mechanisms, consumers tend to behave strategically, either by limiting the amount of information they reveal or by creating multiple accounts and masking their IP address.↩︎